David M. Higdon
- Department Head
Education
- University of Washington, Statistics, Ph.D., 1994
- University of California at San Diego, Mathematics, M.A., 1989
- University of California at San Diego, Mathematics, B.A., 1987
Dave is a professor in the Statistics Department at Virginia Tech. Previously, he spent 14 years as a scientist or group leader of the Statistical Sciences Group at Los Alamos National Laboratory. He is an expert in Bayesian statistical modeling of environmental and physical systems, combining physical observations with computer simulation models for prediction and inference. Dr. Higdon has served on several advisory groups concerned with statistical modeling and uncertainty quantification and co-chaired the NRC Committee on Mathematical Foundations of Validation, Verification, and Uncertainty Quantification. He is a fellow of the American Statistical Association.
- space-time modeling
- inverse problems in hydrology and imaging
- statistical modeling in ecology, environmental science, and biology
- multiscale models
- parallel processing in posterior exploration
- statistical computing
- Monte Carlo and simulation based methods
For a full list of publications, see Dave's Google Scholar Page.
Education
- University of Washington, Statistics, Ph.D., 1994
- University of California at San Diego, Mathematics, M.A., 1989
- University of California at San Diego, Mathematics, B.A., 1987
Dave is a professor in the Statistics Department at Virginia Tech. Previously, he spent 14 years as a scientist or group leader of the Statistical Sciences Group at Los Alamos National Laboratory. He is an expert in Bayesian statistical modeling of environmental and physical systems, combining physical observations with computer simulation models for prediction and inference. Dr. Higdon has served on several advisory groups concerned with statistical modeling and uncertainty quantification and co-chaired the NRC Committee on Mathematical Foundations of Validation, Verification, and Uncertainty Quantification. He is a fellow of the American Statistical Association.
- space-time modeling
- inverse problems in hydrology and imaging
- statistical modeling in ecology, environmental science, and biology
- multiscale models
- parallel processing in posterior exploration
- statistical computing
- Monte Carlo and simulation based methods
For a full list of publications, see Dave's Google Scholar Page.
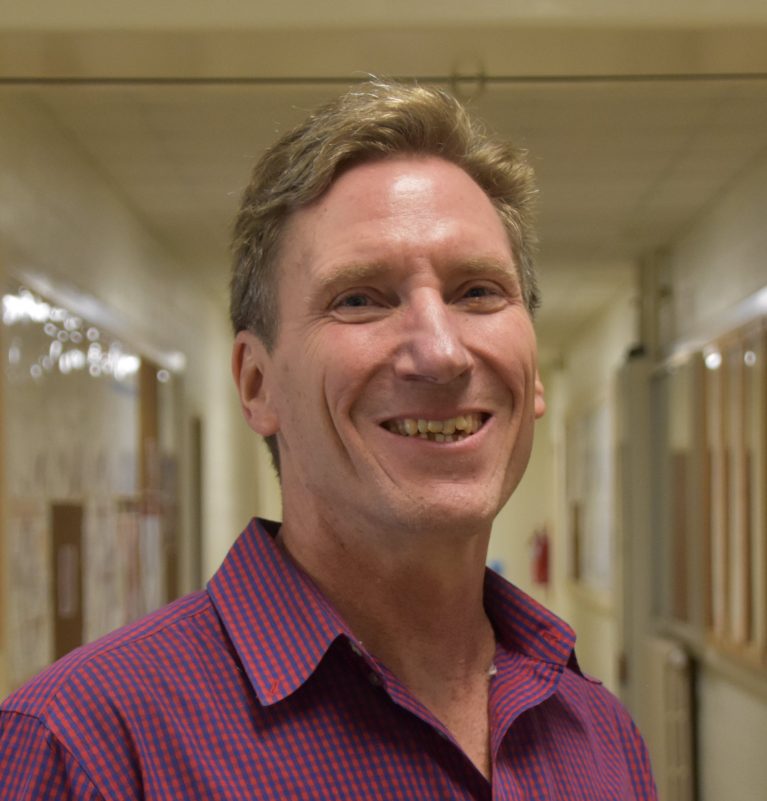
Professor,
Department Head
457 Data and Decision Sciences
727 Prices Fork Road
Blacksburg, Virginia
24061